
__scaled_800.jpg)
In psychology, RL studies show that RL can be hierarchically structured 7 and it interacts with other cognitive functions such as working memory in decision-making 8, 9, 10. RL describes a learning mechanism in which behaviors are shaped through approaching rewards and avoiding punishments, which has a long history dating back to the nineteenth century 6. Reinforcement learning (RL) has made tremendous progress in the past two decades in computer science, psychology and neuroscience 1, 2, 3, 4, 5. Together, we not only show how computational findings from RL align with the spatial navigation literature, but also reveal how the relationship between navigation strategy and a person’s consistency using such strategies changes as navigation requirements change. exploitation tendency (i.e., consistency of using such navigation strategy), which was modulated by navigation task requirements. Furthermore, consistent with a key prediction, there was a correlation in the hybrid model between the weight on model-based learning (i.e., navigation strategy) and the navigator’s exploration vs. Supporting implications from prior literature, the hybrid model provided the best fit regardless of navigation requirements, suggesting the majority of participants rely on a blend of model-free (route-following) and model-based (cognitive mapping) learning in such navigation scenarios. We compared performance of five RL models (3 model-free, 1 model-based and 1 “hybrid”) at fitting navigation behaviors in different phases. One-hundred and fourteen participants completed wayfinding tasks in a virtual environment where different phases manipulated navigation requirements.
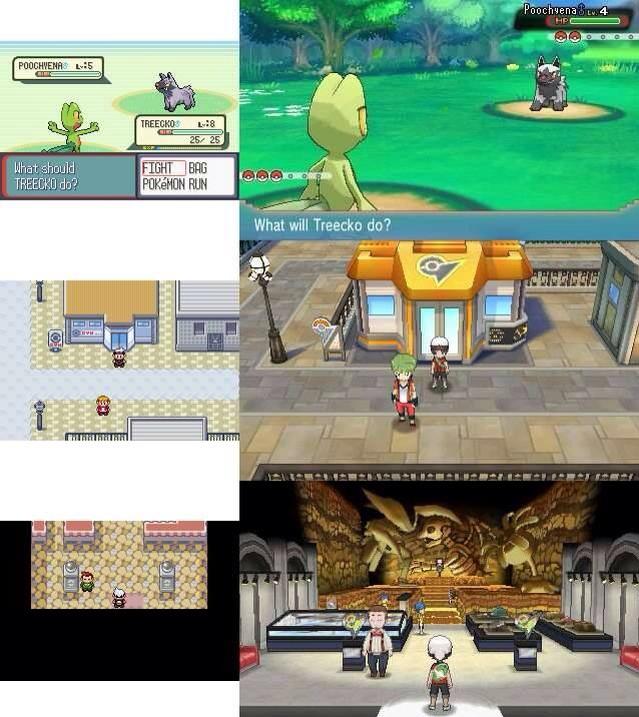
Because RL can characterize one’s learning strategies quantitatively and in a continuous manner, and one’s consistency of using such strategies, it can provide a novel and important perspective for understanding the marked individual differences in human navigation and disentangle navigation strategies from navigation performance. Reinforcement learning (RL) models have been influential in characterizing human learning and decision making, but few studies apply them to characterizing human spatial navigation and even fewer systematically compare RL models under different navigation requirements.
